2024 IT Priorities and Adoption of Gen AI
This document from the Mayfield CXO Network outlines the main IT priorities for 2024, focusing on the integration and adoption of next-generation artificial intelligence.
Mayfield CXO Network January 2024
As we stand on the precipice of 2024, the tech landscape continues to present both exciting opportunities and challenges. Artificial intelligence is starting to push the boundaries of human ingenuity, reshaping industries at an unprecedented pace. In this dynamic environment, IT leaders face the critical task of aligning their AI and IT priorities to navigate the digital frontier and ensure their organizations thrive. This survey delves into the minds of these key decision-makers, exploring their perspectives for the coming year. This year we had 217 responses from IT and innovation leaders across the F2000, we thank you all for your participation. is made up of 2,000+ CIOs, CISOs, CTOs, and Heads of Innovation from companies around the globe who participate in meetings, forums and events with Mayfield to learn about emerging trends and technology. We believe the process of peers learning from one another with the added input of a venture capital perspective is an ideal way to share insights and best practices. Our core belief is to thoughtfully serve this community as a resource, and to provide useful and timely information that benefits our CXO Network members directly in their own roles as leaders. We learn from your input we and hope we’ve gathered peer feedback in a way that is useful to your team in 2024 and beyond. -Gamiel & Shelby
1. AI use cases at this phase are generally internal and experimentative, but . The top three are customer operations, marketing and sales, and software development Over 95% of participants surveyed are interested in buying some or all their gen AI solutions, with “build” primarily justifiable at large organizations with extensive teams already prepared to roll up their sleeves with 56% of surveyed respondents relying on them for deploying gen AI solutions, so not only are companies buying, they’re buying primarily from those most trusted given the technology is still so new , as traditional service providers may not yet have the new talent required. Startups must initially focus on services to get a foot in the door at the enterprise level 6. Overall budgets for IT are less bullish. in many cases
Foreword Key Takeaways Table of Contents 2024: The Gen AI Gold Rush • Early Use Cases • Business Maturity • Buy vs. Build • Deployment • Interesting Solutions Today • Interesting Use Cases Today • Gen AI: The Talk of the Town 2024: IT Budgets • IT Spending • Winners and Losers • AI Outlook 2024: The State of Innovation About Mayfield & How to Engage
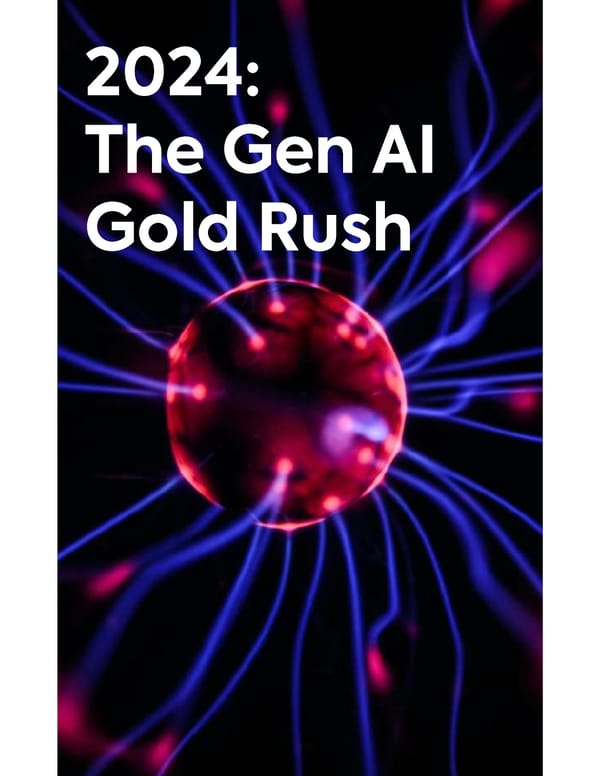
Gen AI is emerging as a powerful new tool for CXO buyers, with the potential to revolutionize the way businesses operate. This is still a relatively new technology, but is rapidly maturing and becoming more accessible to businesses of all sizes. Early adopters will be well- positioned to gain a competitive advantage in the years to come. Today, there’s huge potential for unprecedented opportunity to re- imagine how we work, create, and innovate across a broad swath of industries. We wanted to better understand from our respondents where the promise is for the current moment. On a scale of 1-5 we asked respondents to rate their urgency around the following use cases for gen AI in 2024 (5 = Highly Urgent, 1 = Not Interesting) Customer Operations – Marketing and Sales – Software Engineering – Corporate IT and IT Helpdesk – Product and R&D – Talent and Organization – Risk and Legal – Supply Chain and Operations – Strategy and Finance – According to our responses, the top three surveyed use cases for gen AI going into 2024 are Customer Operations, Marketing and Sales, and Software Engineering, which reflects what other are seeing in the broader market as well. McKinsey is aligned on these results with the caveat that software engineering is the strongest in the tech sector, and customer operations are the weakest in sectors that don’t traditionally need them (e.g. education, pharma, manufacturing, electronics). For at least the next 1-2 years, AI’s role will be primarily assistive (content generation, code completion, enterprise search), not autonomous.
According to McKinsey’s State of AI report in August, the percentage of organizations adopting AI has remained steady at 55%, with many respondents implementing AI in only one or two business functions. This indicates that AI usage today is still limited in scope with considerable untapped potential for value capture in the future. At a high level, AI adoption within large organizations has been following a similar trend to that of cloud and digitization in the past: – Excitement and executive interest around AI’s potential, but no practical experience. – Early trials in narrow use cases, no enterprise strategy – The org structure begins to coalesce; dedicated budget is applied, investments are made in enterprise ML and data – New revenue streams and innovative business models are implemented. AI-driven operations are brought to the next level But all said and done, knowing what the stages of adoption look like won’t help a business advance through them. Assessment is the first step towards progress. Areas of consideration include: – What does your organization’s access to tools, platforms, and infrastructure look like? Are you capable of integrating AI solutions into existing systems and processes? Is your data organized and readily usable? – What are your business objectives and how can AI help contribute to achieving those goals? It’s not just about the use cases, but the ROI, potential risks, actionability, etc. – What will your AI policy look like? This could include categories like data management, privacy, and ethical considerations, as well as establishing roles around AI governance – What are your talent capabilities in terms of AI? Who is in your org today and who could you attract? How could you train or upskill existing employees?
So where were our respondents along the maturity spectrum? We received a balanced mix of responses: - Some gen AI applications in pilot phase (not production) - Some gen AI applications in production - Mostly in the planning stage, no apps in production or pilot - Mostly waiting and seeing before investing resources All in, about two-thirds of our respondents are either in production (in some capacity) or operating pilots today. This about 10% better than McKinsey’s numbers back in August, demonstrating that more and more companies are starting to lean in on AI adoption (or perhaps that this cohort is just a little more engaged). But taking the next steps will require a more strategic approach: – Based on the aforementioned areas of assessment, gaps must be identified and addressed – There should be a 3–5-year strategy in place outlining the organizations AI/ML goals and objectives – In both robust and scalable AI/ML infrastructure as well as in personnel – a dedicated team of professionals will be necessary – Once the roadmap is underway, it’s important to establish clear protocols around governance (policies and best practices) + culture (data-driven decision making) – As AI/ML is integrated into the org’s business processes and product development lifecycle, impact should be closely measured and monitored via robust KPIs
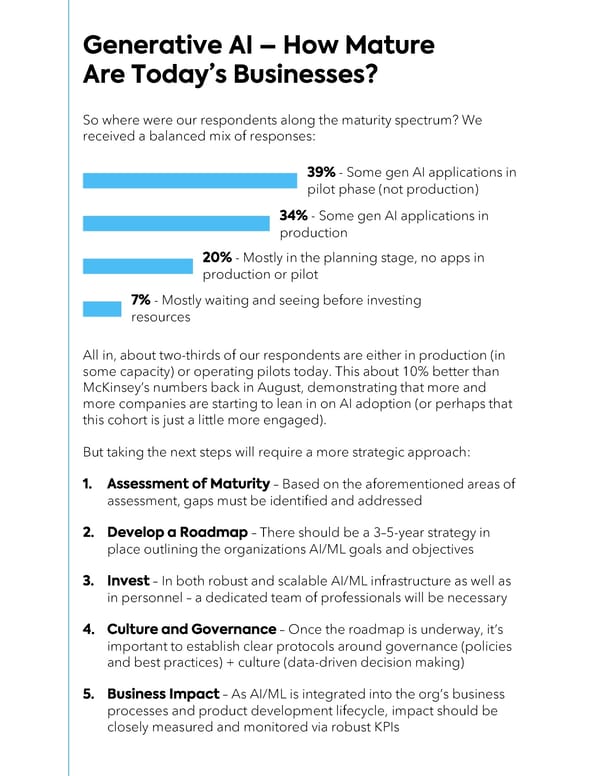
The vast majority of applications will be powered by LLMs inside of the next five years. Organizations today aren’t having too much trouble finding early use cases, but many are at a crossroads when determining how best to proceed. The choice between building and buying holds significant implications for the success of any given application. So, when building out an AI strategy, the first choice is deciding whether to buy vs. build. As foundational LLMs are generally beyond the scope of most companies, we’ll assume that “building” an LLM would involve utilizing an open- source model. The pros and cons are fairly well known today: • Customizable Solution - Can be tailored to specific needs and data. • Greater Control - Over the development process, data ownership, and intellectual property. • Potential Cost Savings - In the long run, especially for large organizations with consistent needs. • Large Initial Investment – Requires significant resources for development and expertise. • Time commitment –Requires dedicated time and personnel for development and maintenance. • Greater Risk of Failure - Requires careful planning and execution to ensure success. , Senior Director (Cyber), AT&T “AI has the potential to revolutionize our productivity and capabilities. The catch will be to build training models with all these different caveats: Do they accurately reflect our needs? Do they introduce unintended biases? Are they secure and locked down? Are the hallucinations eliminated? This requires a deliberate approach with the right levels of governance in place.”
• Faster Time-to-Market - Can access existing solutions and implement them quickly. • Lower Initial Cost - No need for upfront development costs. • Reduced Risk - Leveraging proven solutions with established track records. • Access to Expertise- Benefitting from the knowledge and experience of providers. • Less Customization- May not perfectly fit specific needs and data. • Less Control - Over the data and intellectual property. • Ongoing Costs-Subscription fees or pay-per-use charges. • Vendor Lock-In- Risk of becoming dependent on a specific vendor. • Organizational Size and Resources - Larger organizations with dedicated AI teams are better suited to building. • Technical Expertise - In-house AI expertise is essential for building and maintaining solutions. • Data Availability and Quality - High-quality data is crucial for training generative AI models effectively. • Urgency and Time Constraints- Buying offers a faster solution if time is of the essence. • Budget and Cost Constraints - Building requires a significant upfront investment, while buying involves recurring costs. • Desired Level of Customization - Building allows for tailored solutions, while buying offers standardized options. • Risk Tolerance - Building carries a higher risk of failure, while buying offers a more proven solution. , CIO, Matterport “It’s the right time to make surgical investments that turn the value-add drivers – operational efficiency and/or increased revenue.”
On balance, our respondents were overwhelmingly in favor of “Buy.” - Buy or Build Depending on the Use Case - Buy - Build 96% of respondents want to buy at least some, or in the case of a third of respondents, all of their gen AI solutions, demonstrating that at least for now, there’s safety and convenience in working with the big players. But, as of now, while fewer than 15% of respondents were interested in using solely open-source LLMs (or building their own), two-thirds were interested in experimenting around fine-tuning third party options. When asked whether they want to utilize third party LLMs (e.g. OpenAI or Google) vs. build their own, this is what our respondents had to say: - Will experiment with fine-tuning third-party LLMs + augmenting them with enterprise data - Third party LLMs are good enough - Prefer to pursue open source LLMs - Prefer to build our own enterprise LLMs It’s clear from these early results that there will need to be some combination of third-party LLMs alongside those that are internally hosted and trained, and that this will likely depend on the application. In most cases, third-party will likely make the cut. For example, product- specific help for customers would be easier with a more customizable LLM, but other applications may not require that level of effort. , CIO, BCBC North Dakota “While AI looks to change the way businesses operate, each business still has its own hurdles to address to reach success. This could be data protection, cost to implement, talent, or just determining how to apply AI to the right problem.”
For those looking to build using open-source models, these were the most commonly cited roadblocks: • Lack of clarity around total • Internal talent isn’t cost of ownership and ROI necessarily ready, and • High cost of implementation recruitment is difficult • Sustainable advantage vs. • Service providers are still price and margin erosion evolving to fill the gaps • Security and continuous • Evolving regulatory • Lack of quality data to protection environment power the AI • Lack of appropriate • Fuzzy copyright and • Poor data governance middleware data protections • Data not structured in a • Leakage concerns • Reputational risk and way to deliver • Protection of data + IP liability • No cross-cloud data strategy , CIO, Optomi “Organizations today are rushing to develop gen AI solutions, but if there’s anything I’ve learned in life, it’s that when you rush, you’re more likely to make a mistake. Gen AI is a wonderfully promising and impactful technology, but nothing about it suggests we should set aside the rigor we worked so hard to institutionalize. As we’ve seen in the past with transformative technologies, pressure comes from all sides to be doing something, fast, both for brand and competitive reasons. However seasoned IT leaders know better than to give their processes a hall pass.”
Beyond the top responses, further barriers mentioned were: • Outputs are still • The tools, models, and • Who will own AI unreliable today technology are rapidly initiatives? • Validity of results can evolving, which makes • Where does the budget be questionable it difficult to jump in come from and who will pay? • It’s important to select • Where does this fall vs. • Suboptimal early an architecture that can other pending implementation can balance the trade-offs initiatives? And how lead to low project between performance, much time does it success rates scalability, and require? maintainability • Building AI can mean a • New software delivery • Building trust is slower time to market organizations must be necessary than a more out of the designed to support • Executives need an box solution generative AI appetite to be on the bleeding edge
With their infrastructure, resources, and expertise to provide multi-cloud services, hyperscalers are crucially positioned to capitalize on the promise of generative AI, as they can provide the scalable cloud and storage resources necessary to run these new tools. Furthermore, with recent announcements like Google Gemini, they’re also making critical investments in the space. We wanted to better understand who is currently leading the charge, and where our network is looking to deploy their own gen AI solutions today: - Microsoft - AWS - Google Cloud - Oracle - Cohere, NexGen, or Other Similar Services Based on this survey, Microsoft is still far and away the leader in the space today, with AWS trailing a ways behind. And yet, the future holds many opportunities for competition. opportunities exist in: – Hardware investment in specialized hardware (GPUs, chips, etc.) will accelerate training time and data processing, leading to larger models and more complex datasets – AI-powered enhancements to existing platforms and developer offerings are enabling more efficient deployment of AI models (easier management of backend resources, responses to security incidents, etc.) - GitHub co-pilot from Microsoft, CodeWhispererfrom AWS, and IBM Watson Code Assistant will enable the creation of test cases and test documents, and assist developers in writing clean, efficient code - Hyperscalers are heavily investing in large language models, as cloud computing offers the perfect solution for processing LLMs
Security Note-Taking Custom Apps Ent Search Wearables Marketing Chat Collaboration Presentations Investing rd Vector DB CS Transcription Text to Speech 3 Party LLMs 3D AI Agents Natural Language for Employee Prompt Environments AI App Creation Unstructured Data Support Marketplace LLM App Text to Open-Source Data Framework AI Risk and Builder Image LLMs For LLM Apps Compliance Sales MLOps Eng Copilots , Head of SAP Co-Innovation Desk North America, SAP “Generative AI has unleashed a new era for enterprise applications. It's fundamentally changing how companies operate and we’ll need to focus on addressing the challenges of making AI business-relevant, reliable with trustable processes, and responsible for data privacy and security.”
The general feeling from our respondents is that right now the landscape is changing too quickly, and what was exciting yesterday is not interesting today. There’s a lot of activity going on around security, supply chain, inventory management, customer experience and document summarization/generation (whether these activities are home-grown or not). These are some of the ideas our respondents came back with: • Education – Personalized Learning • Accuracy and Contract Review – 3 Renewals, insurance, other legal • Multi-LLM Frameworks documentation • Order Processing • Agent Platforms – Connecting LLMs • Pharmaceuticals – Drug discovery with actions • Security Operations – Particularly • Agriculture – Coreweave GPUs/CPUs automating end-to-end security tasks • Automation – Development, DevOps, based on gen AI recommendations Security, etc. (e.g. ML for anomaly detection that feeds into gen AI for remediation to • Content Creation – Marketing, sales create an autonomous response) (proposals), legal (boilerplates), security (SOPs). Can be text or visual • Software Development – Including the synthesis of operation signals. AI can • Copilots – Eng, analysts, and more recommend root causes to improve (dataset analysis) • their efficiency • Customer Experience – Chatbot to • Summarization – Lay summaries of chatbot direct interaction, clinical research, manufacturing conversation, and resolution of issues documentation, and more • Customer Predictive Modeling – • Supply Chain – Computer vision, last Forecasting, inventory management mile resilience • Diagnosis – Augmented diagnostic • Test Automation capabilities in healthcare • Videoconferencing , VP Data Science and Analytics, Northwestern Mutual “My favorite perspective on AI comes from historian and storyteller Yuval Noah Harari: ‘AI has hacked the operating system of human civilization.’ Language, rights, religion, and so much more are all cultural artifacts we created by telling stories and writing laws. So what happens when AI becomes better at this than humans?”
Chief Information, Data and Digital Officer, Ally Financial “When used responsibly to create and capture value, generative AI has the ability to impose a generational impact on businesses.” , CIO, Benefix “LLMs may wind up being a great replacement for unsuccessful RPA implementations. So far, we’re seeing a huge opportunity for a fraction of our RPA cost.” , CTO & SVP Product Development, SPROCKIT “Take caution, today, generative AI can be full of misinformation.” , Former CISO, North America, QBE Insurance Group a “AI is another disruptive technology that requires the evaluation of risk and reward in determining where and how it should be used in your organization.” , Head of Digital Product, Engineering & CX, AT&T a “Don't rush for the shiny object: Define the ‘why’ and bring your people together.”
Principal | Deloitte Government & Public Services, Deloitte “A focus on the human experience while designing generative AI solutions will be the key to creating sustainable products with legs.” , CTO, Velocity Global “I expect the delta between the 'Peak of Inflated Expectations' and 'Trough of Disillusionment' to be the smallest of any platform shift we have experienced.” , CIO, Gainsight “Generative AI is over-hyped in the short term, and under-hyped in the long term - the hype cycle is real and has never failed to predict the new technology adoption curve.” , Platforms Head of Data Architecture, Fanatics “Generative AI will shape the next decade with creativity, productivity and intelligence.” , Product Director - Trading Architecture Strategy & Support, Charles Schwab a "We need to shift from just explaining ‘what AI is’ to showcasing ‘what AI can do.’"
Specialist Leader, AI, Deloitte “Generative AI is a paradigm shift in the AI landscape and leads to a democratization of the benefits from this emerging technology.” , Head, Corporate Incubation, TATA Consultancy Services “I believe that AI has the potential to transform people and the planet in a very positive manner.” , Research Director, Commonwealth Cyber Initiative, Research Professor of ECE and Public Policy, Virginia Tech “We have uncovered some surprising applications for LLMs, such as predicting operations. It isn’t all just generating fun poetry.” , CIO, 6Sense “Investing in AI will be critical to staying ahead of your competition. Waiting will immediately put you in the category of laggards.” , President, Kaligent, TGEN "We’re at the beginning of the AI revolution. The real impact of work being done today won't be felt for another five years, and it will be dramatic.”
, President AI (FAIR), Technology and Sustainability, Rackspace “IT leaders must embrace AI across all aspects of IT and usher in a new AI-enabled IT model: Exponential IT.” , Chief Digital and Information Officer, IndiGo “Generative AI will be the difference which will finally make the difference in the world. “ , CTO, Sift “Generative AI will be as innovative and disruptive as the internet and mobile have been. There is a lot of hype right now, but it will in fact alter the IT landscape over the long term.” , SVP Technology,Revature “Generative AI will be a required skill for all professions.” , CISO, Bank of Hope "The CISO role is changing and carries a lot of liability with all the new rules and regulations coming into play. This will force the industry to change the way the organizations protect individuals in these roles, similar to how CFOs are protected today.”
CIO, Exclusive Networks “Generative AI is the largest societal revolution since the launch of the internet and smartphone. Innovation is happening faster and faster which requires executives to step in at the very beginning in order to avoid becoming laggards. Over the next ten years, every company will become an AI company.” , CTO, Ascend Learning “Generative AI represents a significant opportunity to provide organizations with efficiency and productivity in a variety of ways. However, as you think about capabilities in content creation, keeping a human-in-the-loop to review and validate what gets produced is essential for successful implementation of AI- enabled capabilities.” , VP Digital and Data, 24 Hr Fitness “Gen AI is all the rage, with at least three companies per week reaching out to tout their capabilities, integration, and how they can help. Today it feels like there’s a bit more hype than substance and we’re going to take a very cautious approach to utilization and implementation.” , Vice President, Client Delivery, Vation Ventures “I'd expect AI hype to cool off through 2024, while real productivity gains from the technology will be quietly realized in IT operations and software development. Those gains will propel increased investment in the technology in 2025 and beyond.”
COO, PublicRelay “The real limitations on artificial intelligence aren't the boundaries of today's technology, but the limits of our own imagination. AI will transform our world in ways we can't yet fathom, not because we haven't invented the right hardware or algorithms, but because we haven't dared to dream big enough.” , VP Enterprise Business Applications, Emergent BioSolutions “AI is changing at a pace that is unpredictable. In this environment, our greatest tool is our IT organization's ability to understand the value vs the risk, and partner across organizations such as legal, HR, and compliance to develop a comprehensive vision that enables the tech, while protecting enterprise assets.” , CTO, OneMainFinancial “Working with generative AI requires skills familiar to the natural sciences, due to our present inability to explain how it works, and this is going to be hard to grasp for many organizations used to relying on explainability and certainty.” , Director of Data Advisory, BigID “The balance of innovation, regulatory compliance, and customer expectations is the key to progress. Those too conservative (or not conservative enough) may not have to worry about the future. Leadership must define risk vs. lack of innovation, be transparent, ensure alignment, and educate the org to ensure the proper balance of strategy and execution.”
SVP IT, Xilio Therapeutics “An ethical standard should be emplaced in any organization considering the use of AI in an enterprise setting. Once that standard is set, the organization can focus on explainability, interpretability, and, through those, be able to demonstrate fairness and elimination of bias.” , Director, Business Technology and Cybersecurity, Workato “Generative AI use cases to date have had more focus on employee or customer experiences, but 2024 will start to enable digital transformation for IT operations, systems engineering, and security teams with generative AI as the UI layer, and automations acting as the orchestration layer. This will allow these teams to spend more time on AI foundations: including new intake processes, AI-services catalogs, reducing the duplication of AI efforts across the organization, validating models to reduce hallucinations, and holding third party vendors accountable for not only basic SLAs and SLOs, but content AI assurances.” , CIO, IT Director, Snohomish County “The excitement I have seen amongst our traditionally conservative business leaders and elected officials has truly been inspiring and bodes well for our ability to get air under our AI wings in 2024.”
CTO, NIHR Clinical Research Network “To get the maximum value out of generative AI, business and IT leaders must ensure that the use cases are customer-focused, and that trust is established in machine-enabled decision making. This means fundamentally re-evaluating our human decision-making practices, focusing human talent on empathy-based social skills, the contextualization of information and strategy, and creating opportunities for the application of generative AI to data processing and pattern recognition. This will ultimately massively disrupt how we do business. But we must embrace it in order to achieve true digital transformation.” , Former CISO Levi, Cybersecurity Advisor, S3 Consulting “Generative AI is entering the adolescent stage where it’s becoming useful and valuable as an augmentation capability to improve both productivity and customer experience for a wide range of business and consumer use cases. But also like an adolescent, it can be influenced or co-opted in ways that have harmful consequences. So, vigilance must be added as a critical moderating factor to the unbridled enthusiasm of youthful exuberance.” , CIO, Teleperformance “AI will amplify human potential.”
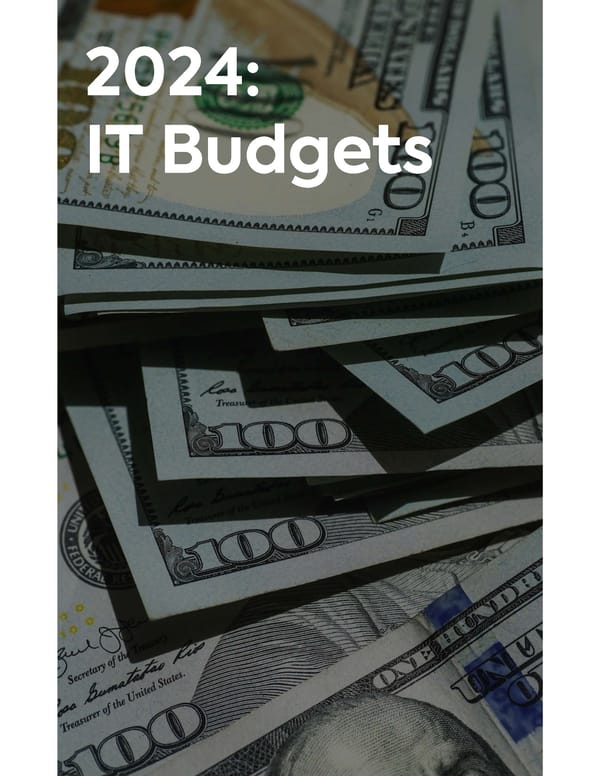
According to Gartner’s latest data, worldwide IT spending is projected to total $5.1 trillion in 2024, an increase of 8.8% from 2022. We asked our network whether or not they agreed with this prediction and how they defined their plans for 2024: : IT has been : We will likely cut elevated as a primary driver of (or have already) our spending plans productivity and will continue to be in a leadership role for 2024 from a budget : Expect standpoint both increases in spending and cost reductions, so a flat overall budget : 2024 will likely be harder economic times for most companies, and all budgets including IT will be scrutinized Our network is aligned with Gartner, with 82% either bullish or tentatively optimistic on budget outcomes for 2024. , with security and sales productivity coming in second and third. The losers are outsourced services, including contractors. Traditional service providers may not yet have the talent required to address complex AI-related needs, leaving an opportunity for startups who focus on services to get a foot in the door at the enterprise level.
Flat Increase Decrease MSSP DevSecOps Anti-Ransomware Trust/Privacy Breach Detection IT Ops and Dev HR/Talent Sales Productivity Finance/Operations Collaboration AI/ML Tooling Gen AI and LLMs Analytics/Visualization Database Consulting Managed Services Contractors
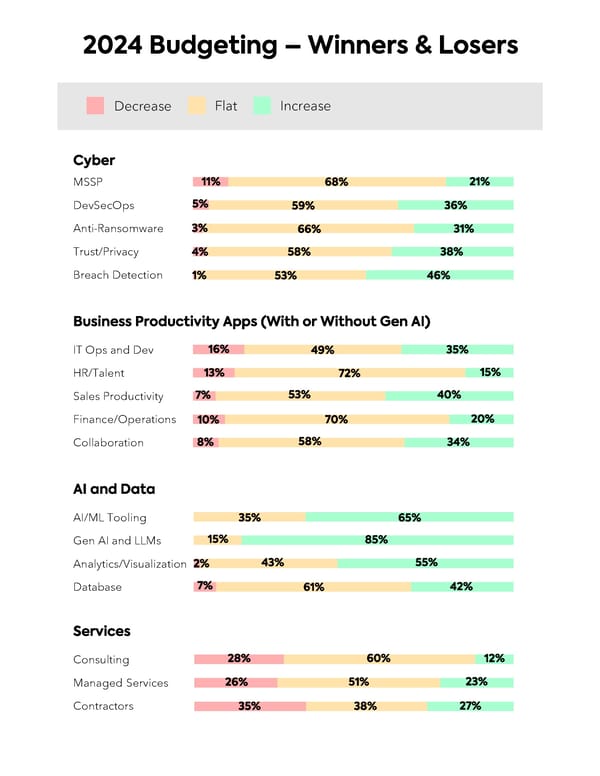
Since generative AI is still a nascent technology, the general sense from Gartner, InfoWorld, and others, is that it will remain a smaller line item in the budget, but greatly increased from before (~3.5X what was originally anticipated). Our respondents offered some areas where they already have definitive budgets in place: - Vendor Solutions from Existing Providers - Gen AI Data Management - Gen AI Evaluation - Gen AI Security - Foundational Model Training and Fine-Tuning - Gen AI Observability - Gen AI Orchestration - Infrastructure to Build Our Own LLM Models - Gen AI Regulation and Trust So right now, existing vendors are leading the charge, with buyers looking to trial additions to their current product lineups (GitHub, Slack, Tableau, Salesforce, etc.). There’s safety in buying from known entities, and for now, the big names are making the largest investments. For those looking to build their own solutions, it’s clear that teams are in the early innings, focused on evaluating early use cases, and organizing/securing their data. Anything more sophisticated than that is being put on the backburner for the time being. , Senior Director, Global IT, IQVIA “Generative AI will be a transformational journey over the next five years. The impact to quality and speed of insights will be invaluable to every industry and will break down silos impeding the creation of new business models. Risks to margins, privacy, and security controls will exist just as they did during the cloud transformation a decade ago, but this will not stop the continuous improvement journey.”
So where are the roadblocks today? We dove into two primary questions with our network around bottlenecks and justification: What are the main bottlenecks for the wider adoption of/budgeting for AI? (5 = Highly Problematic, 1 = Not too problematic) - Concerns About Trust, Safety and Security - Lack of Talent to Build and Deploy Solutions – Data Privacy - Lack of Budgets to Implement Gen AI at Scale - Regulations - Lack of Value to Justify Investment So, the challenges today lie in talent and security, with potential lack of value as the least likely reason respondents came back with. The next question we wanted to understand was how teams are justifying spend in a budget year that may be flat for many teams: What is the primary way your company will justify spending on new generative AI use cases? No new budgets, the money will have to come from existing funding Budgets will mostly from employee productivity savings New budgets will be created based on the gen AI needs of the BUs Costs will be passed to consumers, with superior added functionality Results were somewhat varied, with similar numbers of respondents reporting they’d be expanding budget, displacing budget, or hoping for productivity increases. Most, however, seemed to think it’s too early to pass on costs to customers just yet.
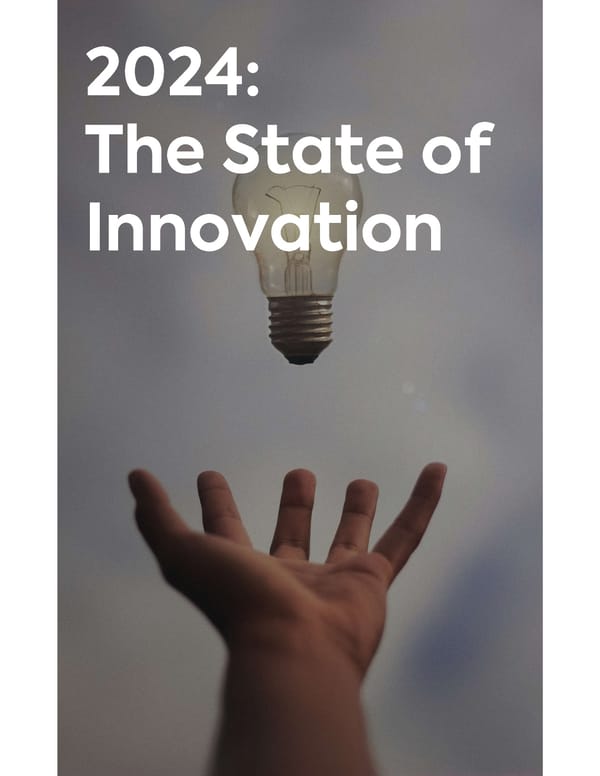
In a year where innovation feels so urgent with the improvements across the AI landscape, we wanted to better understand how the F500 ecosystem was thinking about innovation as a function. In partnership with Peter Temes, Founder & President at the Innovation in Large Organizations Institute, we recently interviewed around 60 innovation leaders focused on guiding change and adding value to their respective large organizations. This was a varied cohort including leaders in CPG (Coca-Cola), Technology (Microsoft), and Healthcare (University of Michigan Health System). Perhaps unsurprisingly, we found a remarkable variety in the level of influence, org structures, and responsibilities of innovation leadership, and far less consistent practice, or even agreement on what best practices are, than we observed five and ten years ago. And yet several major shifts are clear: We see fewer firms establishing or supporting Chief Innovation Officer titles, and more innovation practices landing in operating roles, or shared-services roles like Data and Strategy. Overall, when a Chief Innovation Officer departs a large firm, the most likely replacement is nobody. At the same time, many more mid-level staffers have “innovation” in their job titles. In some cases, this is positive. At firms like AT&T, DFW Airport, and Accenture, innovation as a mindset and mission has been successfully established as part of many people’s jobs, rather than as a special-purpose role. In other cases, the spread of the title is more lip- service than substantial positive change. Most commonly, the role of innovation has begun to consolidate: its core function has become the exploration and deployment of new technology towards existing operations and goals. We expect this phase to last at least five years, at which point there may be a cyclical swing back to business-model and more strategic innovation activities. We conducted solo and group interviews with about 60 innovation leaders, defining the head of innovation as the key executive who has responsibility for the processes that identify, test, and hand-off early- state new products, business models, and operational processes.
or Senior Head of Innovation today than they were five years ago – and yet more innovation activity is alive and growing at lower levels of the org chart. • At one large US-based insurance company, after their Chief Innovation Officer departed, the Chief Digital Officer took over the function, and eventually saw his title change to Chief Digital and Innovation Officer. The vast majority of innovation leaders at large firms rely on existing functional leaders to shape the innovation agenda, and to supply or approve innovation projects. • One innovation leader at a large food and beverage company explained: “I don’t try to sell anything internally.” • A lead innovation executive at SAP shared: “We don’t have appetite for radical or step-change innovation. SAP doesn’t need it…we call [the function] innovation, but it’s more like let’s quickly assess what should be the first proof points and applications of LLMs, as an example.” for innovation leaders across industries, quite dramatically. Understanding and deploying newly maturing technologies – AI and LLMs in particular – has replaced business-model innovation as the top agenda item. , in most of the large organizations we studied. • At a large financial-services firm, the VP of Innovation Development shared: “We are really careful to build a culture that avoids the spotlight and is service oriented. We don’t want to take 12 weeks for a product that’s killed because the interest beyond our team isn’t there.”
5. In most cases, small budgets for core staff and some operating expenses come through annual budgeting processes, (in a few cases, from the strategy function or R&D), with more extended efforts funded by business units. • The head of innovation at a large financial-services firm explained that beyond his core team’s funded HR cost, “getting the buy-in from all the different businesses is the recurring challenge. Any initiative in terms of innovation has to come from the businesses – they have the budget.” , and more likely to have risen up internally, reflecting the shift toward a service-and-exploration agenda, compared to the larger points of emphasis on disruption, business-model change, and organizational change. We found successful leaders clustering into four main groups, as follows: • AXA, Vertiv, Top Build, KPMG, HP (prior to shift), The Coca Cola Company, UM Health System. • HSBC, DFW, Salesforce (new iteration), UAB Medical Center, SAP • Bloomberg, Salesforce (prior to shift), JLL, Microsoft, Harley- Davidson, HTC • Nationwide, Humana, Centene, Vertiv, HSBC , CIO, Chobani “For many of us, 2024 will be a year of cautious experimentation while managing the downside risk of unintended outcomes from pushing too hard, too fast.”
Mayfield is a global venture capital firm with a people-first philosophy and a 50+ year track record of inception and early-stage investing, partnering with founders to build iconic AI, enterprise, semiconductor, and human & planetary health companies. Since its founding, the firm has invested in more than 550 companies, resulting in 120 IPOs and more than 225 acquisitions, and currently has $3B under management. Gamiel Gran & Shelby Golan run the business development team at Mayfield. You can reach out to them at [email protected]& [email protected]. Join us for: • – Themed webinars on pressing topics of the day. 2023 topics included Gen AI: The Enterprise Primer, Gen AI: From Big Vision to Practical Execution, and most recently, An Investor and Practitioner’s Perspective on Gen AI • – 1:1 reviews with you + your team members on key areas of focus, with the goal of sharing new ideas Mayfield has seen that align to your specific use cases • – Regional roundtables with CIOs and other IT executives that are repeated on a semi-annual basis • – https://www.mayfield.com/cxo-of-the-future/ , Former MD, Data & AI, Accenture “Over the last decade, the Mayfield CXO Network has been one of the best sources for me to understand the ongoing business transformations enabled by new technology, and to gain access to cutting-edge innovative startups that help make those transformations real.“